Data inconsistency across systems is a plague that organizations have been trying to purge themselves of since recorded time. With automated and computerized systems all that has happened is that we have switched out clay tablets, coupon counters, and paper-based systems for digital ones, and along the way, we have added more complexity, more data collection points, and more participants to the data collection and management process. Arguably, modern computerized systems have also augmented the audiences for the data that we capture and store, so the breadth of demands made against the data have been elevated, and will continue for the foreseeable future.
As before. data quality, data consistency, and standards play a crucial role in the effective use of the data at our disposal and this is even more critical when we consider the challenges of Golden Nominal creation, particularly when using derivations to combine data from multiple sources.
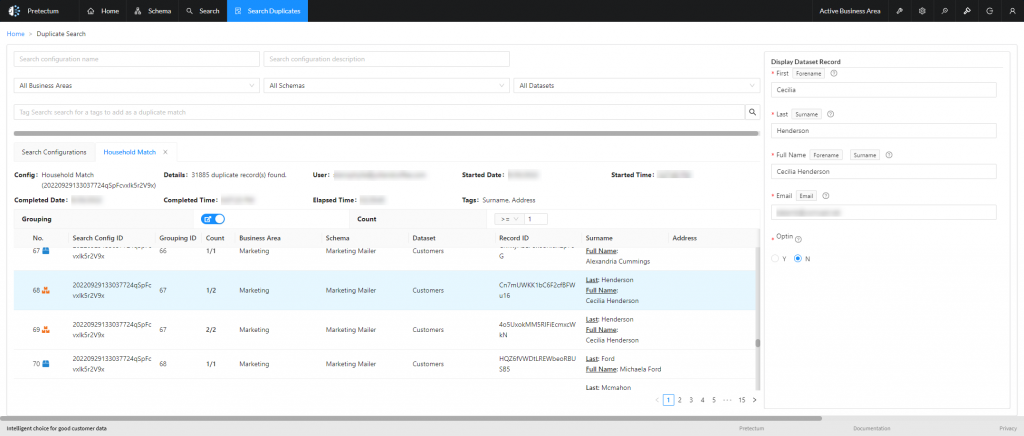
Ensuring Accuracy and Completeness
For the derived Golden Nominal record to be reliable, the underlying data from various sources must be accurate and complete. Any inconsistencies in data quality, such as missing values, incorrect formatting, or outdated information, can lead to an inaccurate or incomplete Golden Nominal record.
When merging data from different systems, conflicts often arise due to variations in data formats, naming conventions, or definitions. Maintaining data consistency is the challenge and achieving this requires robust processes to identify and resolve these conflicts to ensure the derived record accurately represents the most relevant and up-to-date information.
Successful derivation of Golden Nominal records also relies on the effective integration of data from disparate sources. Inconsistencies in their data structures, schemas, or APIs will hinder the integration process and lead to incomplete or misaligned data in the final records.
Maintaining data consistency is crucial for meeting regulatory requirements and adhering to data governance policies and those inconsistencies in the data can lead to non-compliance issues, such as inaccurate reporting or unauthorized data usage, which in turn may result in legal penalties and reputational damage.
Keeping Golden Nominal records up-to-date therefore also requires ongoing maintenance and updates as data changes over time. Consistent data formats, validation rules, and update processes help ensure that the derived records remain accurate and reliable. This is an area where Pretectum CMDM can help.
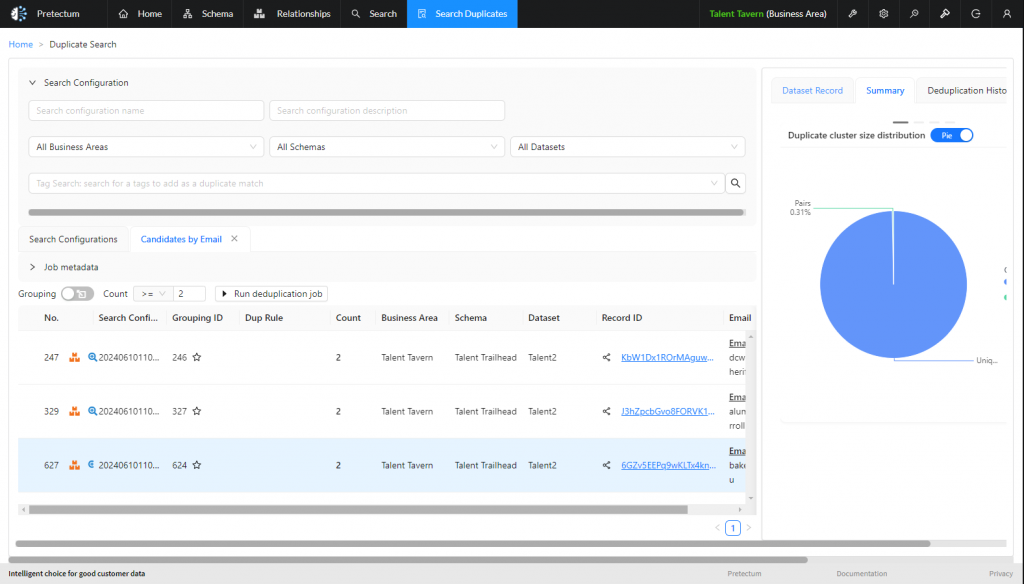
Survivorship vs Derivation
When you start using Pretectum CMDM you may start with pulling data from other systems or importing data via files. This process of data content curation is commonplace. The strength and quality of your schema rules will dictate the baseline data quality parameters. The ETL and data loading and data curation algorithms will manipulate and munge the data into a shape that aligns with the schema rules. This doesn’t address the potentially duplicative nature of the records themselves.
Solving for duplicates is a separate function that deservedly needs specialized rules and handling. The concept of golden nominal establishment is rooted in how any given record lies in the system and how data record singularity and unique records are obtained. Establishment depends on two basic approaches, one of survivorship and one of derivation.
There is no single approach that is guaranteed, nor necessarily preferred, a lot depends on the nature of your business and systems.
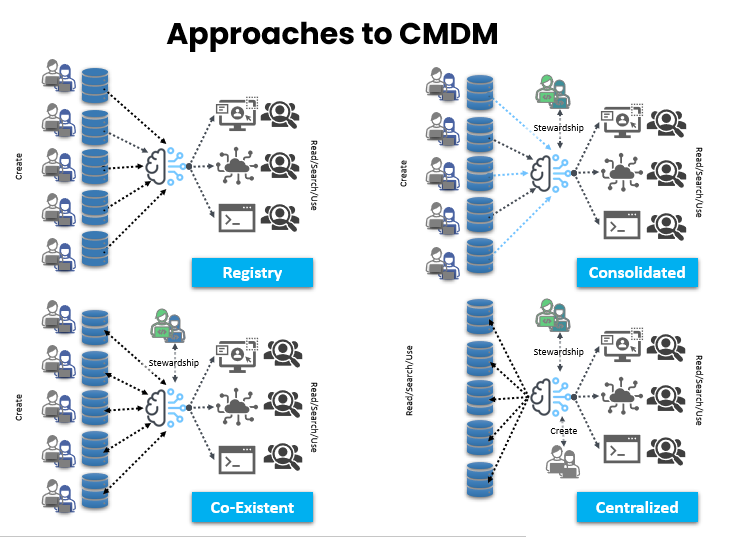
Survivorship
Survivorship involves selecting the most comprehensive record from a set of duplicate records that represent the same entity (e.g., a customer). This is often done by applying a set of rules that compare the values of certain attributes across the duplicate records and choosing the “surviving” record that has the most complete information.
Within Pretectum CMDM this is done through the examination of duplicate record clusters or groupings and the explicit selection of a surviving record. You may nominate, for example, that records coming from your financial systems are the most trusted, the most comprehensive, and the most reliable. As such you may decide that all other systems are subordinate to the financial systems in terms of customer profile definition. Your financial system records of customer profiles would then become, your golden nominal customer system of record. Your single source of customer profile truth (SSOT).
Some common logical approaches for selecting the golden record using survivorship include:
- Manual review and selection
- Prioritizing data sources based on quality scores
- Applying a sequence of rules that compare specific attributes (e.g., choosing the record with the most complete name, then the one with a verified phone number, etc.)
The alternative to survivorship is the derivation of a golden nominal. This could be the physical instantiation of a golden nominal with a persistent record ID or simply the periodic evaluation of all records from all systems, the creation of a golden nominal, and then the syndication and synchronization of the golden nominal attributes with the other systems using their external keys.
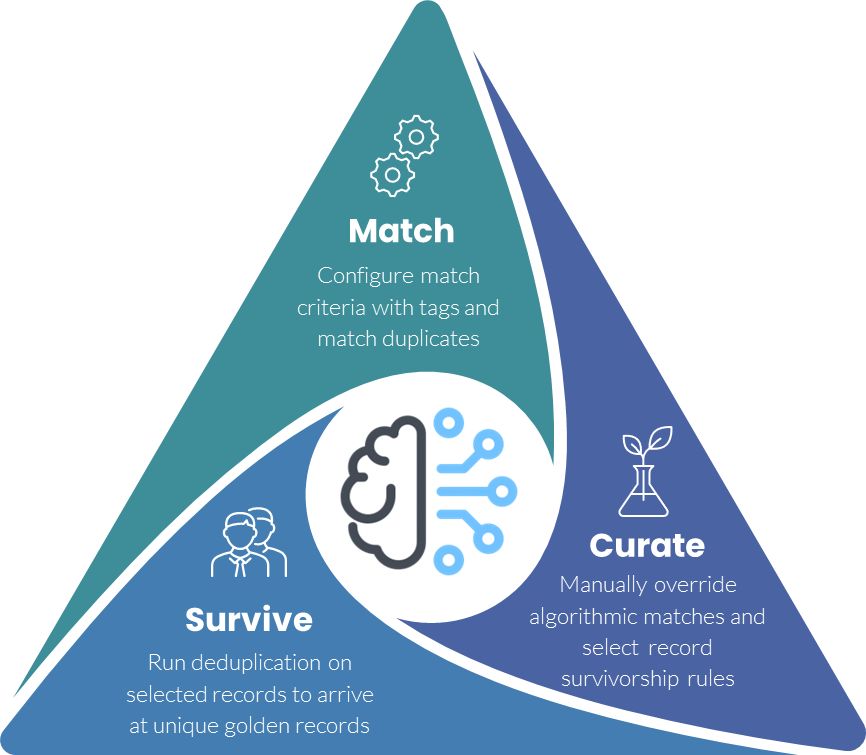
Derivation
Derivation, on the other hand, refers to creating a single, unique reference record by combining information from multiple sources, even if there are no exact duplicate records.
In this case, a golden nominal record is derived by gathering the most accurate and up-to-date information about an individual from various systems and touchpoints within an organization. The goal is to have one true view of the customer where all relevant details are stored in a single place.
Derivation is often used in the public sector to achieve a single view of citizen information, where data is collected across multiple government agencies and systems.
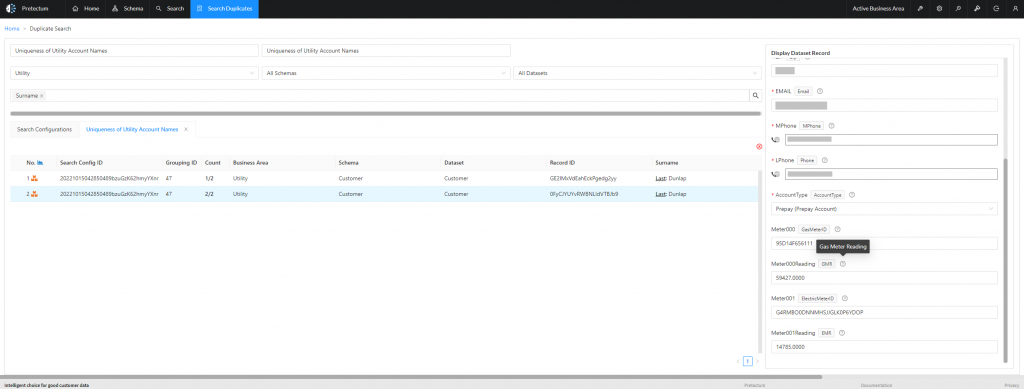
Challenges with Derivation
Using derivation for Golden Nominal creation presents several challenges ranging from data quality through tie-break situations and the overall management of procedure and policy around the nominal.
Derivation relies on combining data from disparate sources, which may have varying levels of accuracy and completeness. Inconsistent data formats, outdated information, or discrepancies in data entry can lead to an unreliable golden nominal record derivation.
Merging data from different systems often involves complex integration. This would include reconciliation between the different data structures, resolving conflicts between overlapping data points, and ensuring that the derived record accurately represents the most relevant information from all sources evaluated.
While derivation aims to create a single view, it can inadvertently result in duplicates. For example, if the same individual is represented in multiple datasets without proper matching logic, more than one record may be established for the same entity. Deduplication processes need to be robust. Careful consideration therefore needs to be given to matching criteria to ensure that the final record is unique.
Data is often subject to change, such as updates to contact information or changes in status which may be impractical to consider in real-time (matching may take some time to complete). Maintaining the golden nominal record requires ongoing updates and monitoring to ensure that it reflects the most current information, which can be resource-intensive especially if a degree of manual scrutiny is also required.
Organizations must establish clear governance policies around the data’s usage, especially when dealing with sensitive personal information. Compliance with privacy regulations adds another layer of complexity to the derivation process, requiring careful management of how data is collected, stored, and utilized so as to ensure that unwanted anomalies, biases, and other factors that might relate to derived data negatively impact the data’s content.
The derivation process can be resource-intensive, particularly in terms of manual oversight effort. It may require significant time and effort to analyze the data alone. While cleaning, and integration from multiple sources can be deterministic and automated, a significant amount of effort may need to be spent on data profiling, algorithms assessment, and rule building. This can strain organizational resources, especially if the data landscape is large and complex and the resources are low.
Contact us to learn more about how Pretectum CMDM can help.