Product recommendations are a critical component of eCommerce personalization strategies, designed to enhance the shopping experience by suggesting products tailored to individual users. Recommendations are generated based on various data inputs, including customer behavior, preferences, and browsing history.
What Are Product Recommendations?
Product recommendations are typically dynamically generated suggestions displayed to users on websites, apps, or emails. They leverage data such as information about the user, demographics and purchase history. Insights gained from the user’s interactions with the site, including products viewed and time spent on specific pages can also be made use of. Factors like location and device type that may influence purchasing decisions so these can be of use also. These recommendations aim to provide a personalized shopping experience, particularly valuable for retailers with extensive and diverse product catalogs.
Types of Product Recommendations
There are several common types of product recommendations used by eCommerce platforms:
- Bestsellers: Highlighting products that are frequently purchased by other customers.
- Trending Products: Showcasing items currently popular among shoppers.
- You Might Also Like: Suggesting products similar to those the user is currently viewing.
- Frequently Bought Together: Items often purchased in combination with basket choices.
Product recommendation systems can be categorized into two main types, those that come from traditional systems that use predefined rules based on historical data (e.g., collaborative filtering). and have limited adaptability to real-time changes and require manual updates; and AI-Powered Systems that use machine learning algorithms to analyze real-time data and customer interactions and which provide dynamic and highly personalized suggestions that improve over time as they learn from user behavior.
Personalized suggestions can boost sales by up to 300% and enhanced targeting can lead to conversion increases of up to 150% with Higher Average Order Value (AOV) recommendations encouraging customers to add more items to their carts,( increasing AOV) having an effect of as much as 50%.
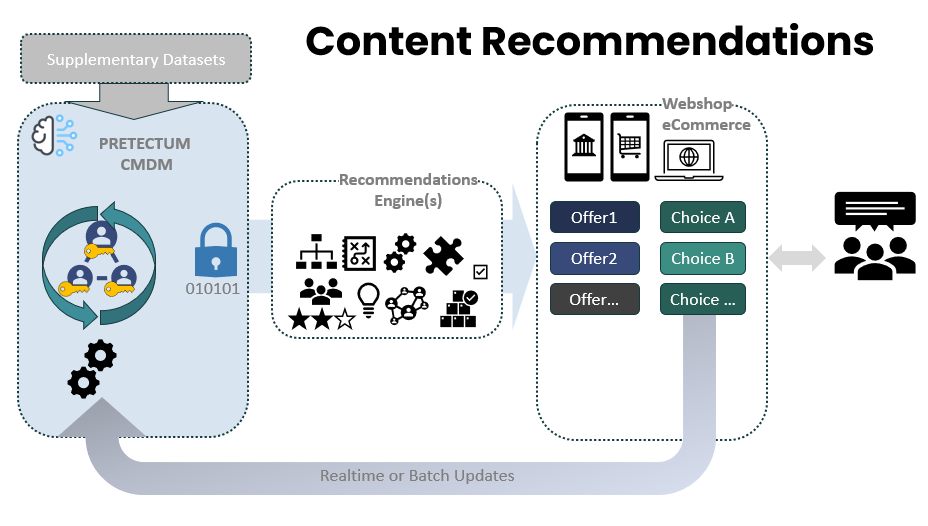
Use Cases with Pretectum CMDM
Pretectum’s Customer Data Management (CMDM) system can significantly enhance product recommendations by leveraging its comprehensive data capabilities. Given that the CMDM has deduplicated customer profiles, often includes demographic data, and can be enriched with additional insights from past purchases and interactions, it can facilitate highly personalized recommendations.
Real-Time Updates
CMDM aggregates extensive information about each customer, including demographics, preferences, and purchase history. This wealth of data allows for more precise targeting when generating product recommendations, ensuring that suggestions align closely with individual customer interests and needs.
Segmentation
By analyzing customer interactions—such as browsing patterns and time spent on specific products, creating profile groups and segments in your analytics platforms and appending this data to the CMDM profiles, your recommendations engines can use this data to support real-time suggestions based on historical and current user behavior, adapting to changes in preferences as they occur.
Pretectum CMDM can serve up the category that a given customer belongs to, as a distinct profile group based on some shared characteristics or behaviors previously supplied and maintained through analysis. Such segmentation allows for tailored marketing strategies and product recommendations that resonate with specific customer segments, improving engagement and conversion rates.
Integration of External Data
The ability to integrate data from various sources enhances CMDM’s effectiveness in providing comprehensive data for recommendations engines. By incorporating this external data from Pretectum into calculations, refine its recommendation algorithms to reflect not only individual customer behavior but also broader market dynamics.
Pretectum’s CMDM can facilitate real-time updates related to the customer to the product recommendations engines based on the most recent customer cross platform interactions indicators that would have been passed to the customer profile . This ensures that the recommendations are not only personalized but also relevant, reflecting current customer interests and preferences.
Behavioral Insights
By providing personalized product suggestions that align with customers’ interests and past behaviors, the CMDM serves to influence customer engagement. This leads to increased satisfaction and loyalty, as customers feel understood and valued through more honed and tailored shopping experiences.
Pretectum CMDM is a powerful tool for organizations that use customer profiles based product recommendations. It does this by harnessing detailed customer data and behavioral insights markers. In support of customer profile segmentation it provides real-time adaptable integration of external data positions which are an essential asset for eCommerce platforms aiming to deliver personalized shopping experiences that drive sales and enhance customer loyalty.