We want to harness the power of Artificial Intelligence (AI) and Machine Learning (ML); after all, it is being pushed into every corner of our lives. It is viewed by futurists as unavoidable. But, just how it will evolve further is still somewhat unclear.
While such cutting-edge technologies are revolutionizing the way businesses analyze large volumes of data, extract meaningful insights, predict customer behaviour, and automate various tasks at its core, humans are still humans. They’re fractious, temperamental and unpredictable. Humans are data contributors and data users and the output of their contributions and behaviours are in use in customer master data management by applications.
While we accept new media into our lives the way we think, what we find appealing, and what we care about may not be entirely different today from generations ago, and generations into the future.
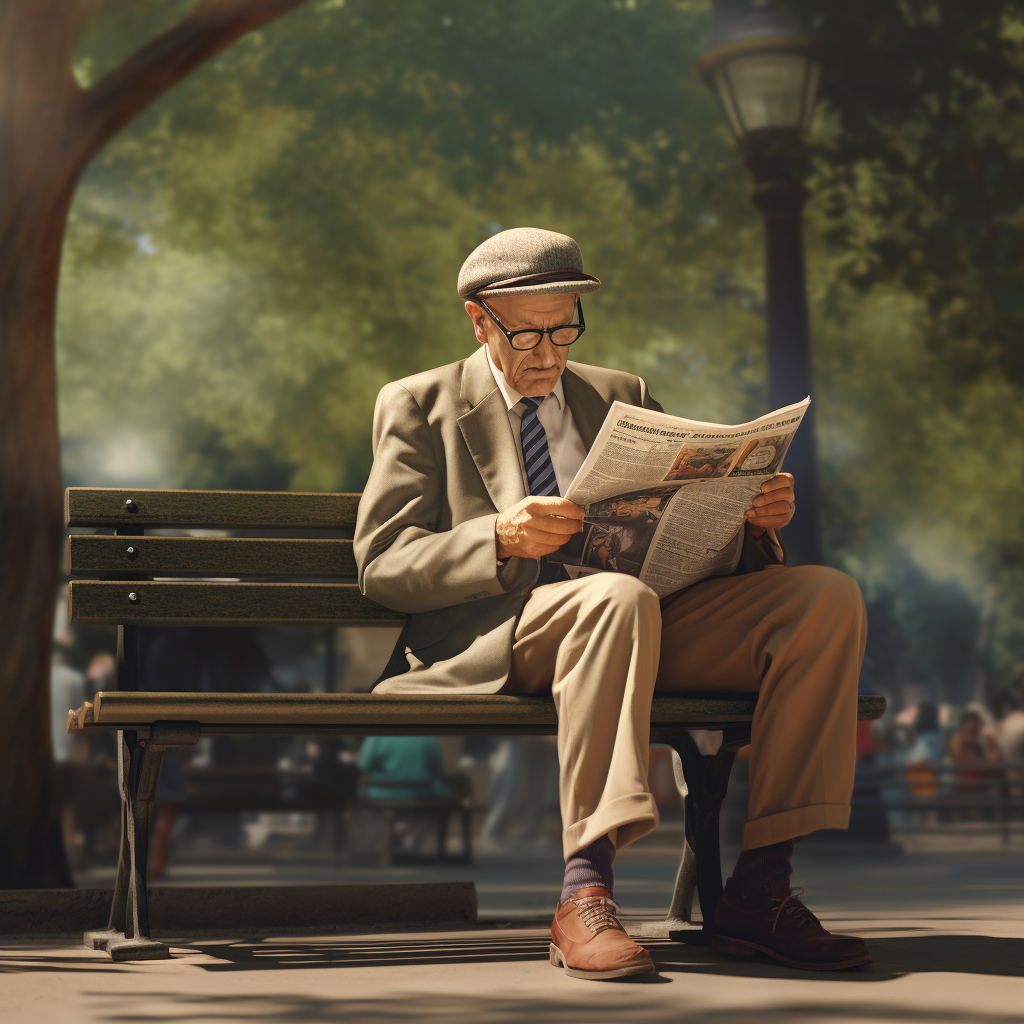
Table talk topics
Integrating Artificial Intelligence (AI) and Machine Learning (ML) in customer data management is great table talk for industry pundits in particular, and undoubtedly triggers lively debate when thought leaders talk about customer data, and data technologies online, in the media and at conferences and trade shows.
While such technologies offer significant benefits, concerns have been raised and remain worrying, about their limitations in truly understanding human behaviours and preferences. I’ve put together and elaborated points, that are perhaps worthy of consideration.
There is substantial evidence supporting the increasing integration of AI and ML in various aspects of our lives, including customer data management in onboarding and engagement journeys, as well as in transacting with business. It is essential to approach the claims of utility with a balanced perspective that considers both the potential benefits and the limitations of these technologies.
At their core, humans are diverse and unpredictable.
AI algorithms in contrast to human thinking and behaviours, have distinct limitations in really predicting human activity due to the complexity and unpredictability of this human nature. Human behaviour is influenced by myriad variables. Those who elaborate and tweak the data models are themselves also tainted with bias and the historical nature of the data itself that is used to train ML and AI models is naturally also biased because it is tied to circumstances that would have been present in the past but may no longer be relevant today.
AI models are just models though, and as such, they lack the real-world experience and contextual understanding of humans in prescribing interaction scripts, suggestions and recommendations. They are trained on patterns in accumulated data, which may limit their ability to comprehend complex social situations that require nuanced understanding.
AI systems also don’t understand language. They look at the statistical correlations of certain word combinations but they have no innate understanding of what even the words themselves mean. This limited understanding of the nuances and subtleties of human language and communication means that models will sometimes serve up completely flawed or inappropriate questions, answers and responses. They are likely to struggle with sarcasm, irony, or figurative language, and cannot understand the context in which language is used, leading to errors or unexpected outcomes.
The immense computing capabilities and high-volume data storage requirements to perform even the most basic AI and ML analysis at scale are also quite far beyond the reach of many organizations so at best. Such businesses have to rely on prebuilt models and algorithms for many of their applications. They may not even have enough of the right kind of data to train home-grown models and most importantly the cost to serve them with custom models may well be very cost-prohibitive or offer a poor return on investment.
We already suggested that bias may be present in the modellers, data scientists and even the data itself and these elevate ethical concerns surrounding the use of AI in significant decision-making processes. Biases in the data often lead to biased outcomes. AI systems most often lack what we consider “common sense” and the necessary transparency of thinking and decision outcomes; i.e. explainability, which are likely to limit their effectiveness in truly understanding human behaviour.
AI cannot authentically simulate human emotions and creativity. Operating based on pre-fed data and past experiences, there is no capacity for creative thinking or genuine emotional understanding, responses are calculated, and scripted with only the smallest of variations over time. without data retraining.
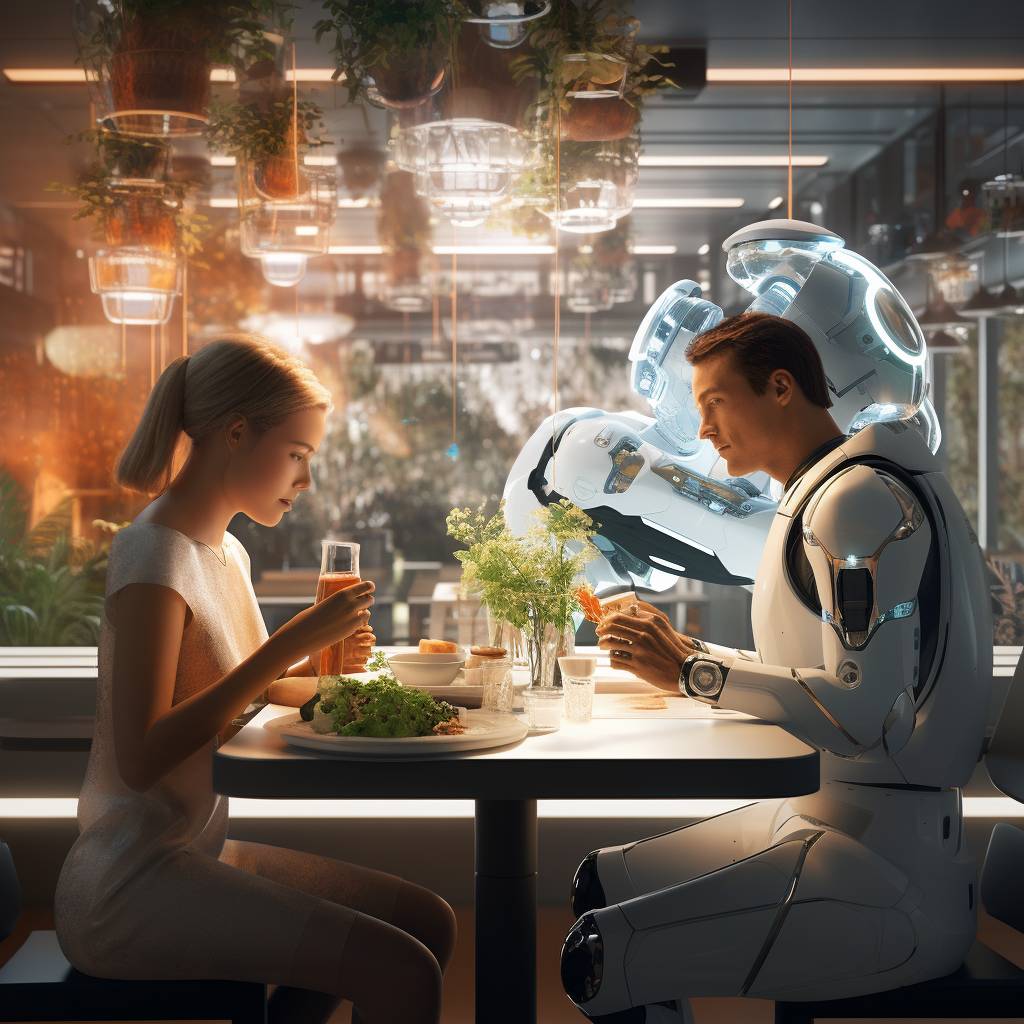
Privacy and ethical concerns
Existing data privacy laws vary from country to country, but they exist to more directly regulate the collection, use, disclosure, cross-border transfer, and other processing of data about identified or identifiable individuals.
Each regulation is jurisdiction-based but gives one a sense of the importance of how you leverage your customer data and might consider the application of AI, and Ml in the markets in which you do business.
For more reading, consider the fifth edition of Global Legal Insights’ (GLI) AI, Machine Learning & Big Data 2023 by contributing editor: Charles Kerrigan is a multi-jurisdictional guide, exploring key legal issues, rules and developments regarding AI, machine learning, and big data across a range of jurisdictions and worth an examination in this context.
Some practical applications
Recent breakthroughs in machine learning are a leap when compared to preceding models and engines but current artificial systems still don’t have the many features of biological intelligence. The complexity of achieving artificial general intelligence (AGI) that matches or exceeds human intelligence remains a major challenge, with significant gaps in flexible, robust, innovative learning, reasoning, and behaviour to be closed.
With all that said, AI and ML can be used to improve customer targeting, campaign optimization, and lead generation in marketing as long as we recognise these limitations and watch for anomalies in the data continuously.
Many companies are incorporating AI and analytics into their customer data management strategies to drive towards better data quality and reach more authentic interactions; and also to help support the development of hyper-personalized recommendations, in pursuit of elevated customer satisfaction.
Data quality and security are paramount concerns in customer data management, and AI plays a significant role in addressing these challenges.
In theory, improved data quality will reduce human error through improved data accuracy.
AI technologies can dramatically improve the practice of customer data management by enhancing accuracy, reducing errors, and ensuring data security. ML algorithms present themselves as powerful tools to potentially enhance data quality by automating various tasks and identifying and rectifying inconsistencies, errors, and missing values in datasets. This not only enhances decision-making processes but also builds trust among stakeholders regarding the integrity of the data being managed.
Keeping data fresh and current is expensive but AI can be of assistance in updating and auto-correcting system data to facilitate businesses staying on top of customer relationship management.
The tooling can track conversations in real-time, and make suggestions based on a bank of prompts or any observed past behaviours where they correlate with the data. By providing feedback to service agents, and enabling the use of AI-driven customer service bots there is some degree of anticipated customer behaviours that might be tied to personalized information exchanges.
When outcomes are prescribed and inputs are clear, unambiguous and standardized, the concept of Autonomous Data Management can be adopted. Here, AI- and ML-powered autonomous data management enables self-service data protection and recovery, freeing up staff to focus on more strategic initiatives. These are effectively automation bound up in the characteristics of AI and ML implementations.
This ADM approach is a double-edged sword since this also introduces the potential for AI to replace human jobs. This raises concerns about potential unemployment and the need for retraining workers for new roles.
Crystal ball gazing
For years, the promise presented by historical data has been the idea that it can be used to predict trends. These trends are often focused on politics, environment and social conditions and then try to anticipate consumer and customer behaviour in response to these variables.
Historical data is used for sales forecasting trends, including seasonal variations, growth patterns, and revenue trends. By analyzing past sales data, businesses make more accurate and reliable sales forecasts, enabling them to plan, allocate, and optimize resources and strategies
Predictive analytics uses historical data to build mathematical models that capture important correlations. These models are then used on current data to predict future events or suggest actions for optimal outcomes. It has applications in various fields such as weather forecasting, economic forecasting, healthcare, engineering, finance, retail, and environmental studies
Making scientific predictions based on historical time-stamped data is referred to as time series forecasts. In business, historical data is used to make forecasts through time series analysis.
This can help in predicting outcomes in areas such as finance, retail, and environmental studies. The state of the forecasting and data makes a difference as to when it should be used. The forecast can be dynamic or static, and the quality of the data is crucial for accurate predictions. They are used in various industries, and the entire point of time series analysis is to facilitate forecasting. It has applications in climate forecasting, economic forecasting, healthcare, engineering, finance, retail, and environmental studies
Various forecasting techniques have also been developed for managerial forecasting problems. The selection of a method depends on the context of the forecast, the relevance and availability of historical data, and the degree of accuracy required. Each technique has its special use, and it is essential to select the correct technique for a particular application.
Final thoughts
The integration of Artificial Intelligence (AI) and Machine Learning (ML) in customer data management presents a potentially transformative landscape, offering both opportunities and challenges to organizations.
While these technologies contribute significantly to data accuracy, security, and automation, their limitations in understanding nuanced human behaviour, potential biases, and the absence of genuine emotional intelligence should be acknowledged.
Recognizing these constraints is crucial for leveraging AI and ML effectively in areas such as customer targeting, campaign optimization, and data quality enhancement.
As this evolving technological frontier develops, ethical considerations, transparency, and a balanced approach are essential to harness the benefits of AI while mitigating potential risks. The path forward involves continuous monitoring, adaptation, and responsible deployment of these advanced tools to ensure they align with our evolving understanding of both technology and humanity.