Customer data has always been critical in aggregate for business decisions. It seems that customer data is becoming increasingly important at the record-entity level as a part of organizational decision-making and also product marketing and development.
Decisions support systems (DSS), need transactional data, yes, but they also need exemplary customer master data in order to inform decision-makers about the audiences that they hope to retain and attract. Data observability is the latest turn of phrase that is getting attention, here are some thoughts on the pertinence with respect to customer master data management.
The question on everyone’s lips will be, whether this requires yet another layer of technology and another layer of data abstraction in order for businesses to be most effective or is this simply an example of vendors pushing a message that promotes product lines that are effectively doing what’s been done before with what is already there?
All indications are that the tool vendors want you to consider data observability in the context of DataOps processes. In other words, with the proliferation of data pipelines that feed various systems, including a CMDM and outbound from CMDM, how are you determining the relative freshness of your customer master data records, where they are being distributed to, the data volume being shuffled about, the variability and consistency from session to session of the schemas, and where the data is coming from, how it is being transformed and manipulated – most commonly understood as lineage.
The arguments in favor of observability are easily understood but not so easily achieved with the plethora of tools and methods that are in use today across IT departments and with the many systems that are in use.
In a nutshell, the call to action is one of, how can you achieve a greater understanding of the current status, quality, reliability, and uptime of data, data exchanges, and data systems. As your business grows and systems proliferate, it is important to be able to confidently answer these questions.
It’s a drum that products like Monte Carlo Data have been beating for a couple of years now and it’s one that you might want to consider as you stitch systems together with ETL and data pipeline processes, including the Pretectum CMDM.
Pretectum and DataOps.
We’re all for a disciplined approach to monitoring and tracking the exchange of data between systems and we recognize that your CMDM is only one of the potentially many cogs in your overall organizational data engine. The good news is that you have potentially many ways of taking stock of the status of your data exchanges and you’re not limited to just what the platform itself offers you out of the box.
Data within your CMDM is constantly changing. Records are created, individually or in bulk and we track and trace every changing job in a verbose history log. This means that reconciliation with tools potentially becomes very valuable because scrutiny by hand, manually, is time-consuming and laborious. While we track data at a detailed level and make this selectively available in the platform we also protect it against prying eyes and technology with a robust set of credential-based authentication methods
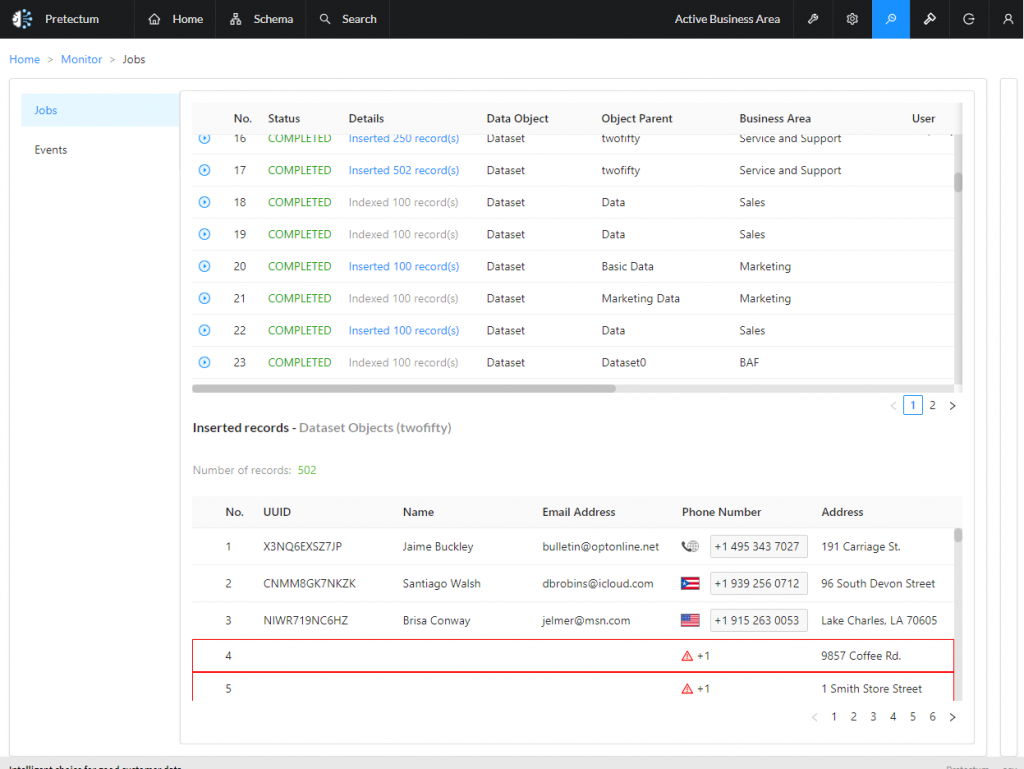
Through the use of APIs in the CMDM data observability can be supported in helping to reconcile data that travels in and out of the Pretectum platform as part of data exchanges and customer master data syndication.
For further information please contact us.